The entries in this blog are really interesting to me AND are selected over Internet.
Friday 29 April 2011
ACM books 04/2011
This is your monthly ACM Technical Interest Service email, providing access to highlights of current ACM activities and information that you can tailor to match your interests. This program is a free ACM Student Membership benefit.
This issue features books from ACM's collection of 500 online titles, powered by Books24/7®. The book offerings have been divided into six "Areas of Specialization," and we have listed the most popular book from each below.
_________________________________________________________________
***Six Most Popular ACM Online Books by Area of Specialization***
1. Area of Specialization:
Web Applications & Services
Most Popular Book:
Cloud Computing: Implementation; Management; and Security
http://learning.acm.org/books/book_detail.cfm?isbn=9781439806807
2. Area of Specialization:
Databases
Most Popular Book:
Practical Guide to Using SQL in Oracle
http://learning.acm.org/books/book_detail.cfm?isbn=9781598220636
3. Area of Specialization:
Programming
Most Popular Book:
Professional Android 2 Application Development
http://learning.acm.org/books/book_detail.cfm?isbn=9780470565520
4. Area of Specialization:
Personal Productivity
Most Popular Book:
175 Ways to Get More Done in Less Time
http://learning.acm.org/books/book_detail.cfm?isbn=9780965878845
5. Area of Specialization:
Office & Productivity Applications
Most Popular Book:
Excel VBA Programming For Dummies; 2nd Edition
http://learning.acm.org/books/book_detail.cfm?isbn=9780470503690
6. Area of Specialization:
Project Management
Most Popular Book:
A Guide to the Project Management Body Of Knowledge (PMBOK® Guide)
http://learning.acm.org/books/book_detail.cfm?isbn=9781933890517
You will receive another message from ACM's Technical Interest Service next month.
The Rise of Hadoop: How many Hadoop-related solutions exist?
The CMSWire commented list of Hadoop-related solutions:
- Apache Hadoop
Appistry CloudIQ Storage Hadoop Edition: a HDFS replacement improving on the single NameNode ( here).
Shipping.
IBM Distribution of Apache Hadoop: Apache Hadoop, a 32-bit Linux version of the IBM SDK for Java 6 SR 8, and an easy-to-use installer that will install and configure both Hadoop (including SSH setup) and Java (here).
Shipping, but in alphaWorks
IBM Global Parallel File System (GPFS): a high-performance shared-disk clustered file system developed by IBM (here).
Shipping.
Cloudera’s Distribution including Apache Hadoop: Cloudera’s packaging for Hadoop and Hadoop toolkit (here).
Shipping.
DataStax Brisk: using Apache Cassandra for Hadoop (and Hive) core services (here).
Announced, but not released yet
Amazon Elastic MapReduce: Amazon hosted Hadoop framework running on the infrastructure of Amazon EC2 and Amazon S3 (here).
Shipping.
Mapr: proprietary replacement for HDFS.
Talked about
CloudStore: the former Kosmos open-source distributed filesystem (here).
Shipping[1]
Pervasive DataRush: parallel data processing optimization for Hadoop jobs (here).
Shipping.
Cascading: query API and query Planner.
Shipping.
Apache Hive: data warehouse on top of Hadoop.
Shipping
Yahoo Pig: high-level data-flow language and execution framework for parallel computation.
Shipping.
Hadapt: hybrid architecture combining relational databases and Hadoop (here).
Announced.
Some others are in the Hadoop toolkit.
Instead of “shipping” another criteria that can be used is number of users and amount of processed data.
Original title and link: The Rise of Hadoop: How many Hadoop-related solutions exist? (NoSQL databases © myNoSQL)
Wednesday 27 April 2011
Wednesday 13 April 2011
Paper: NoSQL Databases - NoSQL Introduction and Overview
Christof Strauch, from Stuttgart Media University, has written an incredible 120+ page paper titled NoSQL Databases as an introduction and overview to NoSQL databases . The paper was written between 2010-06 and 2011-02, so it may be a bit out of date, but if you are looking to take in the NoSQL world in one big gulp, this is your chance. I asked Christof to give us a short taste of what he was trying to accomplish in his paper:
Tuesday 12 April 2011
MapReduce: A major step backwards | The Database Column
MapReduce: A major step backwards
on Jan 17 in Database architecture, Database history, Database innovation posted by DeWitt[Note: Although the system attributes this post to a single author, it was written by David J. DeWitt and Michael Stonebraker]
On January 8, a Database Column reader asked for our views on new distributed database research efforts, and we’ll begin here with our views on MapReduce. This is a good time to discuss it, since the recent trade press has been filled with news of the revolution of so-called “cloud computing.” This paradigm entails harnessing large numbers of (low-end) processors working in parallel to solve a computing problem. In effect, this suggests constructing a data center by lining up a large number of “jelly beans” rather than utilizing a much smaller number of high-end servers.
For example, IBM and Google have announced plans to make a 1,000 processor cluster available to a few select universities to teach students how to program such clusters using a software tool called MapReduce [1]. Berkeley has gone so far as to plan on teaching their freshman how to program using the MapReduce framework.
As both educators and researchers, we are amazed at the hype that the MapReduce proponents have spread about how it represents a paradigm shift in the development of scalable, data-intensive applications. MapReduce may be a good idea for writing certain types of general-purpose computations, but to the database community, it is:
- A giant step backward in the programming paradigm for large-scale data intensive applications
- A sub-optimal implementation, in that it uses brute force instead of indexing
- Not novel at all — it represents a specific implementation of well known techniques developed nearly 25 years ago
- Missing most of the features that are routinely included in current DBMS
- Incompatible with all of the tools DBMS users have come to depend on
First, we will briefly discuss what MapReduce is; then we will go into more detail about our five reactions listed above.
What is MapReduce?
The basic idea of MapReduce is straightforward. It consists of two programs that the user writes called map andreduce plus a framework for executing a possibly large number of instances of each program on a compute cluster.
The map program reads a set of “records” from an input file, does any desired filtering and/or transformations, and then outputs a set of records of the form (key, data). As the map program produces output records, a “split” function partitions the records into M disjoint buckets by applying a function to the key of each output record. This split function is typically a hash function, though any deterministic function will suffice. When a bucket fills, it is written to disk. The map program terminates with M output files, one for each bucket.
In general, there are multiple instances of the map program running on different nodes of a compute cluster. Each map instance is given a distinct portion of the input file by the MapReduce scheduler to process. If N nodes participate in the map phase, then there are M files on disk storage at each of N nodes, for a total of N * M files;Fi,j, 1 ≤ i ≤ N, 1 ≤ j ≤ M.
The key thing to observe is that all map instances use the same hash function. Hence, all output records with the same hash value will be in corresponding output files.
The second phase of a MapReduce job executes M instances of the reduce program, Rj, 1 ≤ j ≤ M. The input for each reduce instance Rj consists of the files Fi,j, 1 ≤ i ≤ N. Again notice that all output records from the map phase with the same hash value will be consumed by the same reduce instance — no matter which map instance produced them. After being collected by the map-reduce framework, the input records to a reduce instance are grouped on their keys (by sorting or hashing) and feed to the reduce program. Like the map program, the reduce program is an arbitrary computation in a general-purpose language. Hence, it can do anything it wants with its records. For example, it might compute some additional function over other data fields in the record. Each reduce instance can write records to an output file, which forms part of the “answer” to a MapReduce computation.
To draw an analogy to SQL, map is like the group-by clause of an aggregate query. Reduce is analogous to theaggregate function (e.g., average) that is computed over all the rows with the same group-by attribute.
We now turn to the five concerns we have with this computing paradigm.
1. MapReduce is a step backwards in database access
As a data processing paradigm, MapReduce represents a giant step backwards. The database community has learned the following three lessons from the 40 years that have unfolded since IBM first released IMS in 1968.
- Schemas are good.
- Separation of the schema from the application is good.
- High-level access languages are good.
MapReduce has learned none of these lessons and represents a throw back to the 1960s, before modern DBMSs were invented.
The DBMS community learned the importance of schemas, whereby the fields and their data types are recorded in storage. More importantly, the run-time system of the DBMS can ensure that input records obey this schema. This is the best way to keep an application from adding “garbage” to a data set. MapReduce has no such functionality, and there are no controls to keep garbage out of its data sets. A corrupted MapReduce dataset can actually silently break all the MapReduce applications that use that dataset.
It is also crucial to separate the schema from the application program. If a programmer wants to write a new application against a data set, he or she must discover the record structure. In modern DBMSs, the schema is stored in a collection of system catalogs and can be queried (in SQL) by any user to uncover such structure. In contrast, when the schema does not exist or is buried in an application program, the programmer must discover the structure by an examination of the code. Not only is this a very tedious exercise, but also the programmer must find the source code for the application. This latter tedium is forced onto every MapReduce programmer, since there are no system catalogs recording the structure of records — if any such structure exists.
During the 1970s the DBMS community engaged in a “great debate” between the relational advocates and the Codasyl advocates. One of the key issues was whether a DBMS access program should be written:
- By stating what you want – rather than presenting an algorithm for how to get it (relational view)
- By presenting an algorithm for data access (Codasyl view)
The result is now ancient history, but the entire world saw the value of high-level languages and relational systems prevailed. Programs in high-level languages are easier to write, easier to modify, and easier for a new person to understand. Codasyl was rightly criticized for being “the assembly language of DBMS access.” A MapReduce programmer is analogous to a Codasyl programmer — he or she is writing in a low-level language performing low-level record manipulation. Nobody advocates returning to assembly language; similarly nobody should be forced to program in MapReduce.
MapReduce advocates might counter this argument by claiming that the datasets they are targeting have no schema. We dismiss this assertion. In extracting a key from the input data set, the map function is relying on the existence of at least one data fi
eld in each input record. The same holds for a reduce function that computes some value from the records it receives to process.
Writing MapReduce applications on top of Google’s BigTable (or Hadoop’s HBase) does not really change the situation significantly. By using a self-describing tuple format (row key, column name, {values}) different tuples within the same table can actually have different schemas. In addition, BigTable and HBase do not provide logical independence, for example with a view mechanism. Views significantly simplify keeping applications running when the logical schema changes.
2. MapReduce is a poor implementation
All modern DBMSs use hash or B-tree indexes to accelerate access to data. If one is looking for a subset of the records (e.g., those employees with a salary of 10,000 or those in the shoe department), then one can often use an index to advantage to cut down the scope of the search by one to two orders of magnitude. In addition, there is a query optimizer to decide whether to use an index or perform a brute-force sequential search.
MapReduce has no indexes and therefore has only brute force as a processing option. It will be creamed whenever an index is the better access mechanism.
One could argue that value of MapReduce is automatically providing parallel execution on a grid of computers. This feature was explored by the DBMS research community in the 1980s, and multiple prototypes were built including Gamma [2,3], Bubba [4], and Grace [5]. Commercialization of these ideas occurred in the late 1980s with systems such as Teradata.
In summary to this first point, there have been high-performance, commercial, grid-oriented SQL engines (with schemas and indexing) for the past 20 years. MapReduce does not fare well when compared with such systems.
There are also some lower-level implementation issues with MapReduce, specifically skew and data interchange.
One factor that MapReduce advocates seem to have overlooked is the issue of skew. As described in “Parallel Database System: The Future of High Performance Database Systems,” [6] skew is a huge impediment to achieving successful scale-up in parallel query systems. The problem occurs in the map phase when there is wide variance in the distribution of records with the same key. This variance, in turn, causes some reduce instances to take much longer to run than others, resulting in the execution time for the computation being the running time of the slowest reduce instance. The parallel database community has studied this problem extensively and has developed solutions that the MapReduce community might want to adopt.
There is a second serious performance problem that gets glossed over by the MapReduce proponents. Recall that each of the N map instances produces M output files — each destined for a different reduce instance. These files are written to a disk local to the computer used to run the map instance. If N is 1,000 and M is 500, the map phase produces 500,000 local files. When the reduce phase starts, each of the 500 reduce instances needs to read its 1,000 input files and must use a protocol like FTP to “pull” each of its input files from the nodes on which the map instances were run. With 100s of reduce instances running simultaneously, it is inevitable that two or more reduce instances will attempt to read their input files from the same map node simultaneously — inducing large numbers of disk seeks and slowing the effective disk transfer rate by more than a factor of 20. This is why parallel database systems do not materialize their split files and use push (to sockets) instead of pull. Since much of the excellent fault-tolerance that MapReduce obtains depends on materializing its split files, it is not clear whether the MapReduce framework could be successfully modified to use the push paradigm instead.
Given the experimental evaluations to date, we have serious doubts about how well MapReduce applications can scale. Moreover, the MapReduce implementers would do well to study the last 25 years of parallel DBMS research literature.
3. MapReduce is not novel
The MapReduce community seems to feel that they have discovered an entirely new paradigm for processing large data sets. In actuality, the techniques employed by MapReduce are more than 20 years old. The idea of partitioning a large data set into smaller partitions was first proposed in “Application of Hash to Data Base Machine and Its Architecture” [11] as the basis for a new type of join algorithm. In “Multiprocessor Hash-Based Join Algorithms,” [7], Gerber demonstrated how Kitsuregawa’s techniques could be extended to execute joins in parallel on a shared-nothing [8] cluster using a combination of partitioned tables, partitioned execution, and hash based splitting. DeWitt [2] showed how these techniques could be adopted to execute aggregates with and without group by clauses in parallel. DeWitt and Gray [6] described parallel database systems and how they process queries. Shatdal and Naughton [9] explored alternative strategies for executing aggregates in parallel.
Teradata has been selling a commercial DBMS utilizing all of these techniques for more than 20 years; exactly the techniques that the MapReduce crowd claims to have invented.
While MapReduce advocates will undoubtedly assert that being able to write MapReduce functions is what differentiates their software from a parallel SQL implementation, we would remind them that POSTGRES supported user-defined functions and user-defined aggregates in the mid 1980s. Essentially, all modern database systems have provided such functionality for quite a while, starting with the Illustra engine around 1995.
4. MapReduce is missing features
All of the following features are routinely provided by modern DBMSs, and all are missing from MapReduce:
- Bulk loader — to transform input data in files into a desired format and load it into a DBMS
- Indexing — as noted above
- Updates — to change the data in the data base
- Transactions — to support parallel update and recovery from failures during update
- Integrity constraints — to help keep garbage out of the data base
- Referential integrity — again, to help keep garbage out of the data base
- Views — so the schema can change without having to rewrite the application program
In summary, MapReduce provides only a sliver of the functionality found in modern DBMSs.
5. MapReduce is incompatible with the DBMS tools
A modern SQL DBMS has available all of the following classes of tools:
- Report writers (e.g., Crystal reports) to prepare reports for human visualization
- Business intelligence tools (e.g., Business Objects or Cognos) to enable ad-hoc querying of large data warehouses
- Data mining tools (e.g., Oracle Data Mining or IBM DB2 Intelligent Miner) to allow a user to discover structure in large data sets
- Replication tools (e.g., Golden Gate) to allow a user to replicate data from on DBMS to another
- Database design tools (e.g., Embarcadero) to assist the user in constructing a data base.
MapReduce cannot use these tools and has none of its own. Until it becomes SQL-compatible or until someone writes all of these tools, MapReduce will remain very difficult to use in an end-to-end task.
In Summary
It is exciting to see a much larger community engaged in the design and implementation of scalable query processing techniques. We, however, assert that they should not o
verlook the lessons of more than 40 years of database technology — in particular the many advantages that a data model, physical and logical data independence, and a declarative query language, such as SQL, bring to the design, implementation, and maintenance of application programs. Moreover, computer science communities tend to be insular and do not read the literature of other communities. We would encourage the wider community to examine the parallel DBMS literature of the last 25 years. Last, before MapReduce can measure up to modern DBMSs, there is a large collection of unmet features and required tools that must be added.
We fully understand that database systems are not without their problems. The database community recognizes that database systems are too “hard” to use and is working to solve this problem. The database community can also learn something valuable from the excellent fault-tolerance that MapReduce provides its applications. Finally we note that some database researchers are beginning to explore using the MapReduce framework as the basis for building scalable database systems. The Pig[10] project at Yahoo! Research is one such effort.
References
[1] “MapReduce: Simplified Data Processing on Large Clusters,” Jeff Dean and Sanjay Ghemawat, Proceedings of the 2004 OSDI Conference, 2004.
[2] “The Gamma Database Machine Project,” DeWitt, et. al., IEEE Transactions on Knowledge and Data Engineering, Vol. 2, No. 1, March 1990.
[4] “Gamma – A High Performance Dataflow Database Machine,” DeWitt, D, R. Gerber, G. Graefe, M. Heytens, K. Kumar, and M. Muralikrishna, Proceedings of the 1986 VLDB Conference, 1986.
[5] “Prototyping Bubba, A Highly Parallel Database System,” Boral, et. al., IEEE Transactions on Knowledge and Data Engineering,Vol. 2, No. 1, March 1990.
[6] “Parallel Database System: The Future of High Performance Database Systems,” David J. DeWitt and Jim Gray, CACM, Vol. 35, No. 6, June 1992.
[7] “Multiprocessor Hash-Based Join Algorithms,” David J. DeWitt and Robert H. Gerber, Proceedings of the 1985 VLDB Conference, 1985.
[8] “The Case for Shared-Nothing,” Michael Stonebraker, Data Engineering Bulletin, Vol. 9, No. 1, 1986.
[9] “Adaptive Parallel Aggregation Algorithms,” Ambuj Shatdal and Jeffrey F. Naughton, Proceedings of the 1995 SIGMOD Conference, 1995.
[10] “Pig”, Chris Olston, http://research.yahoo.com/project/90
[11] “Application of Hash to Data Base Machine and Its
Architecture,” Masaru Kitsuregawa, Hidehiko Tanaka, Tohru Moto-Oka,
New Generation Comput. 1(1): 63-74 (1983)
Friday 8 April 2011
How Facebook Changed Technology in One Day

There are two things I took away from this: (1) by tying the servers and the data center together in a holistic unit, the data center has now officially become computer and (2) the big iron providers have just had the rug pulled out from under them. They will need to shift their business to this data-center centric viewpoint or they will lose out in the very area where their business is growing fastest.
These server systems, which have historically been built by IBM, HP, Dell and now, even Cisco have already begun on a path to consolidation and players such as HP have already been preparing for this future of the data center as a computer by purchasing EYP, a data center design firm. In this vision of the data center as the computer, the server becomes a component, and now, after Facebook’s announcement, it becomes a commodity component of sorts.
Yes, people will still buy servers from Dell, HP and the like, but as more and more people move to on-demand computing either at the infrastructure level as provided by Amazon’s EC2 or at the platform level as provided by an internal cloud or a public PaaS, the older hardware designed for legacy enterprise applications stopS being a growth business. They become mainframes. They’ll still be in the bowels of the building, but it’s not where the newest applications will be built. So the big iron vendors must learn to play a new game for these customers, and it’s a game that Dell is likely the best equipped to play.
Dell doesn’t fret over stripped-down commodity servers — it saw the demand and built out an entire business to sell them called Dell Center Solutions. At the event, Forrest Norrod VP and General Manager of Sever Platforms with Dell, wasn’t worried about the loss to his business from Facebook’s Open Compute efforts, simply saying that Dell will now provide end-to-end solutions and innovation on top of those types of designs. Both HP and Dell were showing off variations of some of the stripped-down, vanity-free Facebook servers and each had borrowed different elements from Facebook while emphasizing how customizable their options were.
In talking to the sales people manning the server prototypes it occurred to me that for webscale customers such as Facebook, it makes sense to put in the time and effort to build your own house, while at the other end there are those who buy EC2 instances or dedicated hosting, which is like renting an apartment. There’s no customization there, a point Jonathan Heiliger the VP of Technical Operations at Facebook, made at the event. However, gear on offer from Cisco, IBM, Dell or HP is like getting a McMansion — there’s some customization, but there are only a few basic models to choose from.
I think in the short-term the market for McMansions is giant as enterprises test out the cloud, but want trusted performance and vendors, but the aspiration for many will be to build their own custom homes. And What Facebook has done is make the custom architecture cheaper to build and run, which in turn makes it easier for other players to come behind and adopt that for better apartments inspired more by the custom homes rather than McMansions.
At the systems level, the news is horribly disruptive, but for the chip companies the pressure is now on. Unlike code, which can be tweaked in a matter of hours or days, hardware has to be built. And if we’re talking about constructing a data center, we’re talking about a construction process that lasts months if not years. So how fast can an open source hardware design iterate? Pat Patla, GM and VP of the server division at AMD, explained that development cycles for hardware and systems have been gradually compressing from 24 months seven or eight years ago to 18 months in the last few years. “Now, lots of folks are comfortable in 12-month development cycles and [Facebook's news] should help more folks get there, but silicon doesn’t move that fast and now there is a very high pressure on the silicon.”
And for those who think this doesn’t change much, I’ll close with Graham Weston, the Chairman of Rackspace ( s rax) who said that Rackspace was planning its next decade of data center projects and had been working on how to build out the right, efficient infrastructure for the last few years. But after it talked to Facebook and saw its 38 percent savings achieved in running the servers he said of the Rackspace plan, “We threw it out.” Now it plans to adapt Facebook’s ideas for its own use, and perhaps build on them.
And that’s the biggest news of all from today’s announcement. Once something is shared with the community, everyone can take part in adding innovation on top of innovation. Today, Facebook has achieved a power usage effectiveness rating of 1.07 which is down from and industry average of 1.5, but now that Facebook has shared, how long until someone can reach .75, or zero? Sure, this is disruptive for the big iron vendors, but it’s also disruptive to the old, slow way of improving our computing infrastructure. So let’s see what’s next.
Related content from GigaOM Pro (subscription req’d):
- Big Data, ARM and Legal Troubles Transformed Infrastructure in Q4
- Infrastructure Overview, Q2 2010
- In Q4, Data Centers, Not the Cloud, Were the Big Story


"
Tuesday 5 April 2011
Introduction to Architecting Systems for Scale
Few computer science or software development programs
attempt to teach the building blocks of scalable systems.
Instead, system architecture is usually picked up on the job by
working through the pain of a growing product
or by working with engineers who have already learned
through that suffering process.
In this post I'll attempt to document some of the
scalability architecture lessons I've learned while working on
systems at Yahoo! and Digg.
- Load Balancing: Scalability & Redundancy
- Caching
- Application Versus Database Caching
- In Memory Caches
- Content Distribution Networks
- Cache Invalidation
- Off-Line Processing
- Platform Layer
I've attempted to maintain a color convention for diagrams in this
post:
- green represents an external request from an external
client (an HTTP request from a browser, etc), - blue represents your code running in some container
(a Django app running on mod_wsgi,
a Python script listening to RabbitMQ, etc), and - red represents a piece of infrastructure (MySQL, Redis, RabbitMQ, etc).
Load Balancing: Scalability & Redundancy
The ideal system increases capacity linearly with adding hardware.
In such a system, if you have one machine and add another, your capacity would double.
If you had three and you add another, your capacity would increase by 33%.
Let's call this horizontal scalability.
On the failure side, an ideal system isn't disrupted by the loss of a server.
Losing a server should simply decrease system capacity by the same amount it increased
overall capacity when it was added. Let's call this redundancy.
Both horizontal scalability and redundancy are usually achieved via load balancing.
(This article won't address vertical scalability,
as it is usually an undesirable property for a large system, as there is inevitably a point
where it becomes cheaper to add capacity in the form on additional machines rather than
additional resources of one machine, and redundancy and vertical scaling can be
at odds with one-another.)
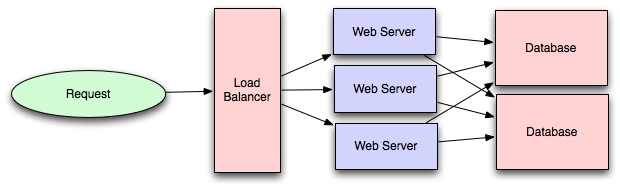
Load balancing is the process of spreading requests across multiple resources according
to some metric (random, round-robin, random with weighting for machine capacity, etc)
and their current status (available for requests, not responding, elevated error rate, etc).
Load needs to be balanced between user requests and your web servers,
but must also be balanced at every stage to achieve full scalability and redundancy
for your system. A moderately large system may balance load at three layers: from the
- user to your web servers, from your
- web servers to an internal platform layer, and from your
- internal platform layer to your database.
There are a number of ways to implement load balancing in your setup.
Smart Clients
Adding load-balancing functionality into your database (cache, service, etc) client
is usually an attractive solution for the developer. Is it attractive because it is
the simplest solution? Usually, no. Is it seductive because it is the most robust? Sadly, no.
Is it alluring because it'll be easy to reuse? Tragically, no.
Developers lean towards
smart clients because they are developers, and so they are used to writing software to
solve their problems, and smart clients are software.
With that caveat in mind, what is a smart client? It is a client which takes a pool
of service hosts and balances load across them, detects downed hosts and avoids
sending requests their way (they also have to detect recovered hosts, deal with adding
new hosts, etc, making them fun to get working decently and a terror to get working correctly).
Hardware Load Balancers
The most expensive--but very high performance--solution to load balancing is to buy a dedicated hardware
load balancer (something like a Citrix NetScaler). While they can solve a remarkable range of problems,
hardware solutions are remarkably expensive, and they are also 'non-trivial' to configure.
As such, generally even large companies with substantial budgets will often avoid using dedicated hardware for
all their load-balancing needs; instead they use them only as the first point of contact from user
requests to their infrastructure, and use other mechanisms (smart clients or the hybrid approach discussed
in the next section) for load-balancing for traffic within their network.
Software Load Balancers
If you want to avoid the pain of creating a smart client,
and purchasing dedicated hardware is excessive,
then the universe has been kind enough to provide a hybrid approach: software load-balancers.
HAProxy is a great example of this approach.
It runs locally on each of your boxes, and each service you want to load-balance
has a locally bound port. For example, you might have your platform machines accessible
via
localhost:9000
, your database read-pool at localhost:9001
and your databasewrite-pool at
localhost:9002
. HAProxy manages healthchecks and will remove and returnmachines to those pools according to your configuration, as well as balancing across all
the machines in those pools as well.
For most systems, I'd recommend starting with a software load balancer and moving to
smart clients or hardware load balancing only with deliberate need.
Caching
Load balancing helps you scale horizontally across an ever-increasing
number of servers, but caching will enable you to make vastly better
use of the resources you already have, as well as making otherwise
unattainable product requirements feasible.
Caching consists of: precalculating results (e.g. the number of visits from each referring domain for the previous day),
pre-generating expensive indexes (e.g. suggested stories based on a user's click history), and
storing copies of frequently accessed data in a faster backend (e.g. Memcache instead of PostgreSQL.
In practice, caching is important earlier in the development process than load-balancing,
and starting with a consistent caching strategy will save you time later on.
It also ensures you don't optimize
access patterns which can't be replicated with your caching mechanism or access patterns where performance becomes
unimportant after the addition of caching
(I've found that many heavily optimized Cassandra applications
are a challenge to cleanly add caching to if/when the database's caching strategy can't
be applied to your access patterns, as the datamodel is generally inconsistent between the Cassandra
and your cache).
Application Versus Database Caching
There are two primary approaches to caching: application caching and
database caching (most systems rely heavily on both).
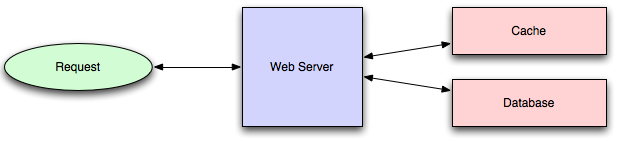
Application caching requires explicit integration in the application code itself.
Usually it will check if a value is in the cache; if not, retrieve the value from
the database; then write that value into the cache (this value is especially
common if you are using a cache which observes the least recently used caching algorithm).
The code typically looks like (specifically this is a read-through cache, as it
reads the value from the database into the cache if it is missing from the cache):
key = "user.%s" % user_id user_blob = memcache.get(key) if user_blob is None: user = mysql.query("SELECT * FROM users WHERE user_id=\"%s\"", user_id) if user: memcache.set(key, json.dumps(user)) return user else: return json.loads(user_blob)
The other side of the coin is database caching.
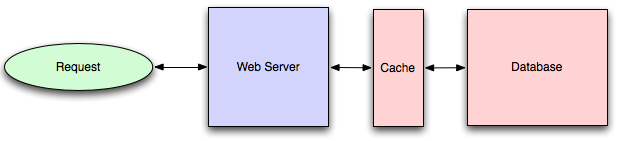
When you flip your database on, you're going to get some level
of default configuration which will provide some degree of caching and performance.
Those initial settings will be optimized for a generic usecase,
and by tweaking them to your system's access patterns you can generally
squeeze a great deal of performance improvement.
The beauty of database caching is that your application code gets faster 'for
free', and a talented DBA or operational engineer can uncover
quite a bit of performance without your code changing a whit
(my colleague Rob Coli spent some time recently optimizing our configuration for Cassandra
row caches, and was succcessful to the extent that he spent a week harassing us with graphs
showing the I/O load dropping dramatically and request latencies
improving substantially as well).
In Memory Caches
The most potent--in terms of raw performance--caches you'll encounter are those which store
their entire set of data in memory. Memcached and
Redis are both examples of in-memory caches (caveat: Redis can be configured
to store some data to disk).
This is because accesses to RAM are orders of magnitude
faster than those to disk.
On the other hand, you'll generally have far less RAM available than disk space, so you'll
need a strategy for only keeping the hot subset of your data in your memory cache. The most
straightforward strategy is least recently used, and is employed by Memcache (and Redis as of 2.2 can
be configured to employ it as well). LRU works by evicting less commonly used data in preference of
more frequently used data, and is almost always an appropriate caching strategy.
Content Distribution Networks
A particular kind of cache (some might argue with this usage of the
term, but I find it fitting) which comes into play for sites serving large amounts
of static media is the content distribution network.
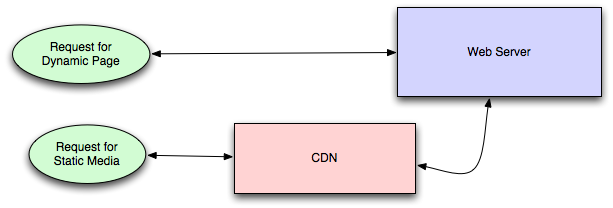
CDNs take the burden of serving static media off of your application servers (which are
typically optimzed for serving dynamic pages rather than static media), and
provide geographic distribution. Overall, your static assets will load more quickly
and with less strain on your servers (but a new strain of business expense).
In a typical CDN setup, a request will first ask your CDN for a piece of static media, the
CDN will serve that content if it has it locally available (HTTP headers are used for configuring
how the CDN caches a given piece of content). If it isn't available, the CDN will query your
servers for the file and then cache it locally and serve it to the requesting user (in this
configuration they are acting as a read-through cache).
If your site isn't yet large enough to merit its own CDN, you can ease a future transition
by serving your static media off a separate subdomain (e.g.
static.example.com
) usinga lightweight HTTP server like Nginx, and cutover the DNS from
your servers to a CDN at a later date.
Cache Invalidation
While caching is fantastic, it does require you to maintain consistency between your
caches and the source of truth (i.e. your database), at risk of truly bizarre applicaiton behavior.
Solving this problem is known as cache invalidation.
If you're dealing with a single datacenter, it tends to be a straightforward
problem, but it's easy to introduce errors if you have multiple codepaths writing to your
database and cache (which is almost always going to happen if you don't go into writing the application
with a caching strategy already in mind). At a high level, the solution is: each time a value changes,
write the new value into the cache (this is called a write-through cache)
or simply delete the current value from the cache and allow a read-through cache to populate it later
(choosing between read and write through caches depends on your application's details,
but generally I prefer write-through caches as they reduce likelihood of a stampede
on your backend database).
Invalidation becomes meaningfully more challenging for scenarios involving fuzzy queries (e.g if you
are trying to add application level caching in-front of a full-text search engine like
SOLR), or modifications to unknown number of elements
(e.g. deleting all objects created more than a week ago).
In those scenarios you have to consider relying fully on database caching, adding aggressive
expirations to the cached data, or reworking your application's logic to avoid the issue
(e.g. instead of
DELETE FROM a WHERE...
, retrieve all the items which match the criteria,invalidate the corresponding cache rows and then delete the rows by their primary key explicitly).
Off-Line Processing
As a system grows more complex, it is almost always necessary to perform processing
which can't be performed in-line with a client's request either because it is creates
unacceptable latency (e.g. you want to want to propagate a user's action across a
social graph) or it because it needs to occur periodically (e.g. want to create daily rollups
of analytics).
Message Queues
For processing you'd like to perform inline with a request but is too slow,
the easiest solution is to create a message queue (for example, RabbitMQ).
Message queues allow your web applications to quickly publish messages to the queue,
and have other consumers processes perform the processing outside the scope and timeline
of the client request.
Dividing work between off-line work handled by a consumer and in-line work done by
the web application depends entirely on the interface you are exposing to your users.
Generally you'll either:
- perform almost no work in the consumer (merely scheduling a task)
and inform your user that the task will occur offline, usually with a polling mechanism
to update the interface once the task is complete
(for example, provisioning a new VM on Slicehost follows this pattern), or - perform enough work in-line to make it appear to the user that the task has completed,
and tie up hanging ends afterwards (posting a message on Twitter or Facebook likely
follow this pattern by updating the tweet/message in your timeline but updating
your followers' timelines out of band; it's simple isn't feasible to update all
the followers for a Scobleizer
in real-time).
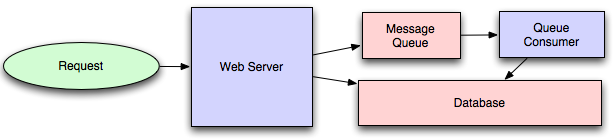
Message queues have another benefit, which is that they allow you
to create a separate machine pool for performing off-line processing
rather than burdening your web application servers. This allows you
to target increases in resources to your current performance or throughput bottleneck
rather than uniformly increasing resources across the bottleneck and non-bottleneck
systems.
Scheduling Periodic Tasks
Almost all large systems require daily or hourly tasks,
but unfortunately this seems to still be a problem waiting
for a widely accepted solution which easily supports redundancy.
In the meantime you're probably still stuck with cron,
but you could use the cronjobs to publish messages to a consumer,
which would mean that the cron machine is only responsible for scheduling
rather than needing to perform all the processing.
Does anyone know of recognized tools which solve this problem?
I've seen many homebrew systems, but nothing clean and reusable.
Sure, you can store the cronjobs in a Puppet
config for a machine, which makes recovering from losing that machine
easy, but it would still require a manual recovery, which is probably
acceptable but not quite perfect.
Map-Reduce
If your large scale application is dealing with a large quantity of data,
at some point you're likely to add support for map-reduce,
probably using Hadoop, and maybe
Hive or HBase.
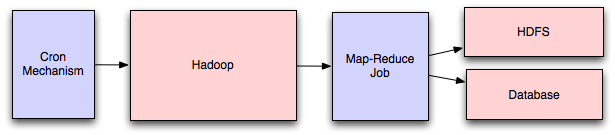
Adding a map-reduce layer makes it possible to perform data and/or processing
intensive operations in a reasonable amount of time. You might use it for calculating
suggested users in a social graph, or for generating analytics reports.
For sufficiently small systems you can often get away with adhoc queries
on a SQL database, but that approach may not scale up trivially once the
quantity of data stored or write-load requires sharding your database,
and will usually require dedicated slaves for the purpose of performing
these queries (at which point, maybe you'd rather use a system designed
for analyzing large quantities of data, rather than fighting your database).
Platform Layer
Most applications start out with a web application communicating
directly with a database. This approach tends to be sufficient
for most applications, but there are some compelling reasons
for adding a platform layer, such that your web applications communicate
with a platform layer which in turn communicates with your databases.
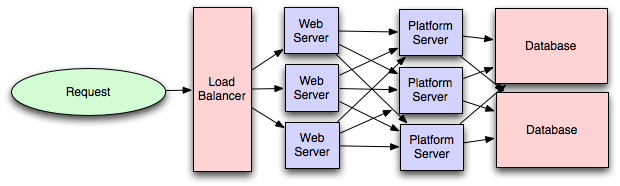
First, separating the platform and web application allow you to scale
the pieces independently. If you add a new API, you can add platform servers
without adding unnecessary capacity for your web application tier.
(Generally, specializing your servers' role opens up an additional level
of configuration optimization which isn't available for general purpose
machines; your database machine will usually have a high I/O load and will
benefit from a solid-state drive, but your well-configured application server probably
isn't reading from disk at all during normal operation, but might benefit from
more CPU.)
Second, adding a platform layer can be a way to reuse your infrastructure for multiple
products or interfaces (a web application, an API, an iPhone app, etc) without writing
too much redundant boilerplate code for dealing with caches, databases, etc.
Third, a sometimes underappreciated aspect of platform layers is that they
make it easier to scale an organization. At their best, a platform exposes a crisp product-agnostic
interface which masks implementation details.
If done well, this allows multiple independent teams to develop utilizing the platform's
capabilities, as well as another team implementing/optimizing the platform itself.
I had intended to go into moderate detail on handling multiple data-centers, but
that topic truly deserves its own post, so I'll only mention that cache
invalidation and data replication/consistency become rather interesting problems
at that stage.
I'm sure I've made some controversial statements in this post,
which I hope the dear reader will argue with such that we can
both learn a bit. Thanks for reading!
"
Sunday 3 April 2011
Presentation:Parallel Programming Patterns: Data Parallelism
Friday 1 April 2011
NoSQL & Cloud at Netflix
Today Netflix can be seen as a leader in what can be achieved by combining cloud computing and polyglot persistence. Not only that, but Netflix has chosen to share their experience with everyone else so we can all learn from their experience.
Netflix’s experience of migrating from an on-premise architecture using relational databases has been documented over time. Here are a couple of important points in the history of migrating from the classical architecture to the mostly in the cloud solution they are currently using and continuing to experiment and build:
- Challenges of a Hybrid solution: Oracle - Amazon SimpleDB
- Practical tips for optimizing Amazon SimpleDB access
- Netflix’s transition to High-Availability storage systems
- Why Netflix picked Amazon SimpleDB, Hadoop/HBase, and Cassandra
- The key technical challenge of cloud computing
- What sort of data can you move to NoSQL?
- Which NoSQL technologies are we working with?
- How did we translate RDBMS concepts to NoSQL?
Original title and link: NoSQL & Cloud at Netflix (NoSQL databases © myNoSQL)